This PoC demonstrates enhanced traffic monitoring and fine granular telemetry streaming solutions for optical access networks. In other words, the PoC focuses on the use-case of traffic monitoring and data analytics and demonstrates a full Machine Learning (ML) pipeline comprising telemetry retrieval from data sources (i.e., Optical Line Terminal (OLT)), telemetry broker, real time write/read to/from Time Series Databases (TSDB), telemetry consumption by the ML models for traffic forecasting, and eventually the ML-assisted solution. The key contributions are fourfold: 1) definition and development of a full telemetry streaming procedure for PONs, which includes the mapping between a PON structure and the functional blocks of the framework (e.g., broker, database, inference host) and their interfaces; 2) development of a ML-assisted traffic forecasting model that follows traffic variations in the order of 10-15 seconds with high accuracy; 3) incorporation of a ML inference host to the telemetry workflow for real-time prediction and analysis; and 4) implementation of a cloud-native version of the whole pipeline in which every individual block runs in a dedicated Docker container that allows easier deployment and automation. The live demonstration has been carried out on the F5G OpenLab testbed.
The developed solution complies with the specifications laid down in the telemetry work item of the European Telecommunication Standards Institute (ETSI) Industry Specifications Group (ISG) Fifth Generation Fixed Network (F5G).
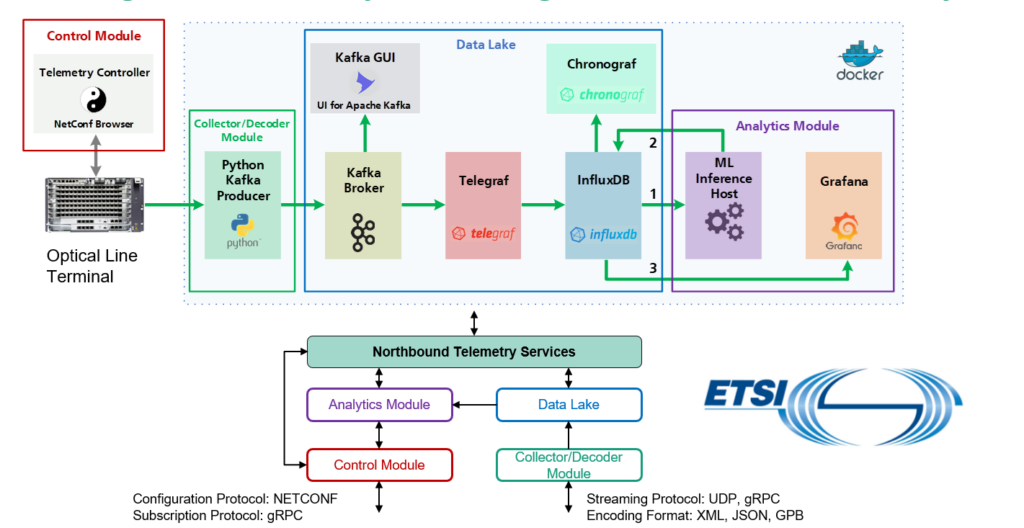